AIって結局何なのかよく分からないので、とりあえず100日間勉強してみた Day2
経緯についてはこちら
NumPy User Guide を読んでみた #1 はこちら
■本日の進捗
●NumPy User Guide を理解
■Numpyの使い方で調べたこと
前回に続いてNumPy User Guideを読んでみたので気になった点を挙げて理解を深めていきます。
●Slicingについて
恐らく超基礎項目から。
Data[0:2]はIndex0からIndex2までのことなのかな?と思ったが、どうやら「Index0から2つ」という意味らしい。
Data[-2:]に関しては Index-2 はそもそも存在しない(いや、数値を返すんだから存在するのか… 何だか複素数みたいだな)。「Index2を含む」と考えた方が理解が良いかもしれない。
●nonzeroはゼロでないインデックスを返す
>>> import numpy as np
>>> arr = np.array([[0, 1, 0],[4, 0, 5]])
>>>
>>> indices = np.nonzero(arr)
>>> print(indices)
(array([0, 1, 1]), array([1, 0, 2]))
これは一見意味がわからないが、次のように考えるとわかりやすい。
array([[0, 1, 0],
[4, 0, 5]])
ゼロでない場所のインデックスを意識してみよう。
最初の 1 は0行1列目に存在する。(インデックスは通常0から始まる)
次の 4 は1行0列目に、その次の 5 は1行2列目にそれぞれ存在する。
これをまとめて書けば、下記のようになる。
行インデックス: array([0, 1, 1])
列インデックス: array([1, 0, 2])
あらびっくり、nonzeroの返り値と一緒になりましたね。
●Hsplitの意味
ぱっと見意味不明だが、これも一度理解すれば何てことはないと思います。
>>> x = np.arange(1, 25).reshape(2, 12)
>>> x
array([[ 1, 2, 3, 4, 5, 6, 7, 8, 9, 10, 11, 12],
[13, 14, 15, 16, 17, 18, 19, 20, 21, 22, 23, 24]])
>>>
>>> np.hsplit(x, (3, 4))
[array([[ 1, 2, 3],
[13, 14, 15]]), array([[ 4],
[16]]), array([[ 5, 6, 7, 8, 9, 10, 11, 12],
[17, 18, 19, 20, 21, 22, 23, 24]])]
これはつまり、xを分割するのに、まず3列目(Index0〜2)までで分割。
次に4列目(Index3)を分割して、残りは何の指定もないので区切ることなく出力すれば上記の通りになる。
●flattenとravel
要点のみ。
np.flattenは、元の配列のコピーを作成し、1次元の配列を返す。
np.ravelは、元の配列のビューを返す(コピーを作成することもある)。
flattenは常に新しいコピーを作成するが、ravelは可能ならビューを返すためメモリ効率が良い。
●help関数について
最も重要にして最も有用と言っても過言ではないhelp関数だが、使うには少々癖がある。help関数の使い方(各項目の1行目)に注目してください。
maxの場合
maxはPythonの組み込み関数(Python標準のbuilt-in functions)なので、
help(max)
Help on built-in function max in module builtins:
max(…)
max(iterable, *[, default=obj, key=func]) -> value
max(arg1, arg2, *args, *[, key=func]) -> value
With a single iterable argument, return its biggest item. The
default keyword-only argument specifies an object to return if
the provided iterable is empty.
With two or more arguments, return the largest argument.
(END)
sortの場合
sortはリストオブジェクトのメソッド(Pythonのリスト型オブジェクトに対して直接呼び出すことができる関数)なので、
help([].sort)
Help on built-in function sort:
sort(*, key=None, reverse=False) method of builtins.list instance
Stable sort *IN PLACE*.
(END)
transposeの場合
transposeはNumPy配列のメソッド(numpy.ndarrayに対して直接呼び出すことができる関数)なので、
help(np.ndarray.transpose)
Help on method_descriptor:
transpose(…)
a.transpose(*axes)
Returns a view of the array with axes transposed.
For a 1-D array this has no effect, as a transposed vector is simply the
same vector. To convert a 1-D array into a 2D column vector, an additional
dimension must be added. `np.atleast2d(a).T` achieves this, as does
`a[:, np.newaxis]`.
For a 2-D array, this is a standard matrix transpose.
For an n-D array, if axes are given, their order indicates how the
axes are permuted (see Examples). If axes are not provided and
``a.shape = (i[0], i[1], ... i[n-2], i[n-1])``, then
``a.transpose().shape = (i[n-1], i[n-2], ... i[1], i[0])``.
Parameters
----------
axes : None, tuple of ints, or `n` ints
* None or no argument: reverses the order of the axes.
* tuple of ints: `i` in the `j`-th place in the tuple means `a`'s
`i`-th axis becomes `a.transpose()`'s `j`-th axis.
* `n` ints: same as an n-tuple of the same ints (this form is
intended simply as a "convenience" alternative to the tuple form)
Returns
-------
out : ndarray
View of `a`, with axes suitably permuted.
See Also
--------
ndarray.T : Array property returning the array transposed.
ndarray.reshape : Give a new shape to an array without changing its data.
Examples
--------
>>> a = np.array([[1, 2], [3, 4]])
>>> a
array([[1, 2],
[3, 4]])
>>> a.transpose()
array([[1, 3],
[2, 4]])
>>> a.transpose((1, 0))
array([[1, 3],
[2, 4]])
>>> a.transpose(1, 0)
array([[1, 3],
:
確認したい関数がどんなオブジェクトに対する関数なのかを意識して呼び出さないと確認ができないので注意したい。使いこなせればもはやこれだけでいいくらい無敵になれる…はず。
以下、ChatGPT大先生が教えてくださったので掲載。
まあ、都度調べればいいと思う。
Pythonリストオブジェクトのメソッド一覧
- append()
- extend()
- insert()
- remove()
- pop()
- clear()
- index()
- count()
- sort()
- reverse()
- copy()
NumPy配列のメソッド一覧
- T(転置プロパティ)
- transpose()
- flatten()
- ravel()
- reshape()
- resize()
- astype()
- copy()
- view()
- fill()
- sum()
- prod()
- mean()
- std()
- var()
- min()
- max()
- argmin()
- argmax()
- cumsum()
- cumprod()
- sort()
- argsort()
- searchsorted()
- clip()
- repeat()
- choose()
- nonzero()
- where()
- take()
- compress()
- diagonal()
- trace()
- dot()
- matmul()
- conj()
- real()
- imag()
- transpose()
- trace()
- mean()
- std()
- var()
MeanSquareErrorについて
User Guideに言及があったので調べてみた。
Mean Square Error (MSE) は、回帰モデルの予測性能を評価するための指標で、scikit-learnにもライブラリがあったので使ってみた。
>>> from sklearn.metrics import mean_squared_error
>>> y_test = [1, 2, 3, 4]
>>> y_pred = [1.5, 2.5, 3.5, 4.5]
>>> mse = mean_squared_error(y_test, y_pred)
>>> mse
0.25
これをUser Guideにある通りにNumPyのみでやってみると下記の通り。
>>> y_test = np.array([1, 2, 3, 4])
>>> y_pred = np.array([1.5, 2.5, 3.5, 4.5])
>>> error = (1/4) * np.sum(np.square(y_test - y_pred))
>>> error
0.25
もちろん答えは同じ。この程度だと記述に関しては特に変わりがないが、やっぱりライブラリとして使えた方が便利だと思う一方で、自分で書いてみると何をしているのかよく理解できるからこのくらいなら自分で手を動かすのもいいかなと思った。
●NumPy配列を保存する
こちらも要点のみ。
np.savetxt, np.loadtxtは配列をテキストデータで扱う。
np.save, np.loadはdata, shape, dtype等の情報を保持したNumPyバイナリ形式(.npy)で扱う。
np.savezはzipファイルのように複数の配列を1つのデータとして保存でき、拡張子は.npzになる。
使い方はこんな感じ。
np.save(‘filename’, ‘arr’) #拡張子を.csvと明示することも可能
load_data = np.load(‘filename.npy’)
np.genfromtxtはテキストデータに欠損値や数値以外のデータ型がある場合に有効で、コメントアウトも回避する他、データ型の自動推定や任意に指定することもできる。
data = np.genfromtxt(
'data.txt',
delimiter=',',
dtype=[('col1', 'i4'), ('col2', 'f4'), ('col3', 'U10')],
missing_values='NaN', # 欠損値として 'NaN' を指定
filling_values=np.nan # 欠損値を np.nan で埋める
)
■おわりに
とりあえずNumPy v2.1のユーザーガイドは一通り目を通して全て理解できました。「Pythonではじめる機械学習」にはこの後、SciPy, matplotlib, pandasと紹介が続いていくのですが、SciPyがちょっと重そう(雰囲気的に?)なので、明日はpandasから理解していこうかなと思っています。そもそも著者の勧めに従ってSciPy Lecture Notesに手をつけないで、勝手にNumPyユーザーガイドから始めたのでもう順番とかいいかなって…笑
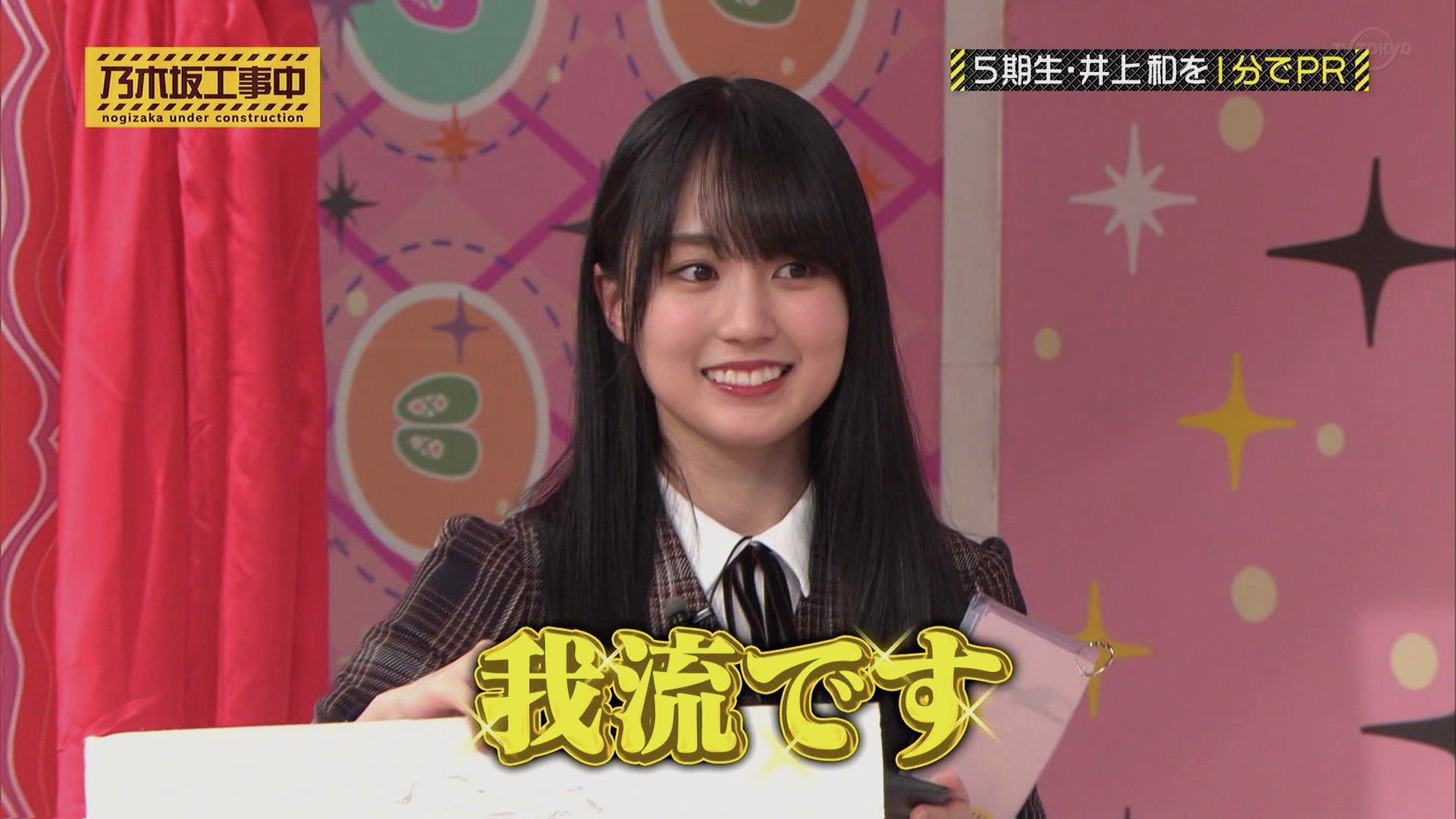
■参考文献
- NumPy user guide – NumPy v2.1 Manual. numpy.org. 2024.
https://numpy.org/doc/2.1/user/index.html - ChatGPT. 4o mini. OpenAI. 2024.
https://chatgpt.com/