AIって結局何なのかよく分からないので、とりあえず100日間勉強してみた Day80
経緯についてはこちらをご参照ください。
■本日の進捗
- CBoWを理解
■はじめに
今回も「ゼロから作るDeep Learning② 自然言語処理編(オライリー・ジャパン)」から学んでいきます。
今回は、前回実装したバイアスのない全結合層や関数を用いてCBoWを実装していきます。
■簡易的なCBoW
CBoWはone-hot処理関数とMatMul層によって、one-hot encordingされた入力ベクトルXiを行列Wによって埋め込みに変換した結果をViとすると、下記のようにベクトルを得ます。
$$ \mathrm{\boldsymbol{V}}_i = \mathrm{\boldsymbol{X}}_i \mathrm{\boldsymbol{W}}^{\boldsymbol{T}} $$
このベクトルを平均化し、全体の情報を1つのベクトルに集約したベクトルを得ます。
$$ \mathrm{\boldsymbol{h}} = \frac{1}{2C} \sum_{j=-C}^C \mathrm{\boldsymbol{V}}_{i+j} $$
このベクトルhを用いて、各単語のスコアを算出します。
$$ \mathrm{\boldsymbol{z}} = \mathrm{\boldsymbol{W}}’^{\mathrm{T}} \mathrm{\boldsymbol{h}} $$
このスコアzをSoftmaxによって確率分布に変換したら、交差エントロピー誤差によって損失を計算するというニューラルネットワークで良く見てきた形になります。(実装するこれらの層も流用できます。)
ここまでの流れをSimpleCBoWというクラスで実装すれば下記のようになります。
class SimpleCBoW: def __init__(self, vocab_size, hidden_size): V, H = vocab_size, hidden_size W_in = 0.01 * np.random.randn(V, H).astype('f') W_out = 0.01 * np.random.randn(H, V).astype('f') self.layers = [ MatMul(W_in), MatMul(W_out) ] self.loss_layer = SoftmaxCrossEntropy() self.params, self.grads = [], [] for layer in self.layers: self.params += layer.params self.grads += layer.grads self.word_vecs = W_in def forward(self, contexts, target): h0 = self.layers[0].forward(contexts[:, 0]) h1 = self.layers[0].forward(contexts[:, 1]) h = (h0 + h1) * 0.5 score = self.layers[1].forward(h) loss = self.loss_layer.forward(score, target) return loss def backward(self, dout=1): ds = self.loss_layer.backward() da = self.layers[1].backward(ds) da *= 0.5 self.layers[0].backward(da) return None
■CBoWの学習
上記の簡易的なCBoWを用いて簡単なコーパスを学習させてみます。最適化アルゴリズムにはAdamを使用しますが、もちろんこれも画像認識の場合のニューラルネットワークと全く同じものが使えます。
学習ループに関しても基本的にはこれまでの手法を踏襲しているので新しいことはありません。
import numpy as np import matplotlib.pyplot as plt class MatMul: def __init__(self, W): self.params = [W] self.grads = [np.zeros_like(W)] self.x = None def forward(self, x): W, = self.params out = np.dot(x, W) self.x = x return out def backward(self, dout): W, = self.params dx = np.dot(dout, W.T) dW = np.dot(self.x.T, dout) self.grads[0][...] = dW return dx class SoftmaxCrossEntropy: def __init__(self): self.output = None self.y_true = None self.loss = None def forward(self, logits, y_true): exp_values = np.exp(logits - np.max(logits, axis=1, keepdims=True)) self.output = exp_values / np.sum(exp_values, axis=1, keepdims=True) self.y_true = y_true self.loss = -np.sum(y_true * np.log(self.output + 1e-7)) / y_true.shape[0] return self.loss def backward(self): return (self.output - self.y_true) / self.y_true.shape[0] def cross_entropy_error(y, t): delta = 1e-7 return -np.sum(t * np.log(y + delta)) / y.shape[0] class Adam: def __init__(self, lr=0.001, beta1=0.9, beta2=0.999): self.lr = lr self.beta1 = beta1 self.beta2 = beta2 self.iter = 0 self.m = None self.v = None def update(self, params, grads): if self.m is None: self.m, self.v = {}, {} for key, val in params.items(): self.m[key] = np.zeros_like(val) self.v[key] = np.zeros_like(val) self.iter += 1 lr_t = self.lr * np.sqrt(1.0 - self.beta2 ** self.iter) / (1.0 - self.beta1 ** self.iter) for key in params.keys(): self.m[key] = self.beta1 * self.m[key] + (1 - self.beta1) * grads[key] self.v[key] = self.beta2 * self.v[key] + (1 - self.beta2) * (grads[key] ** 2) params[key] -= lr_t * self.m[key] / (np.sqrt(self.v[key]) + 1e-7) def preprocess(text): text = text.lower() text = text.replace('.', ' .') words = text.split(' ') word_to_id = {} id_to_word = {} for word in words: if word not in word_to_id: new_id = len(word_to_id) word_to_id[word] = new_id id_to_word[new_id] = word corpus = np.array([word_to_id[w] for w in words]) return corpus, word_to_id, id_to_word def convert_one_hot(corpus, vocab_size): N = corpus.shape[0] if corpus.ndim == 1: one_hot = np.zeros((N, vocab_size), dtype=np.int32) for idx, word_id in enumerate(corpus): one_hot[idx, word_id] = 1 elif corpus.ndim == 2: C = corpus.shape[1] one_hot = np.zeros((N, C, vocab_size), dtype=np.int32) for idx_0, word_id in enumerate(corpus): for idx_1, word_id in enumerate(word_id): one_hot[idx_0, idx_1, word_id] = 1 return one_hot def create_contexts_target(corpus, window_size=1): target = corpus[window_size:-window_size] contexts = [] for idx in range(window_size, len(corpus)-window_size): cs = [] for t in range(-window_size, window_size + 1): if t == 0: continue cs.append(corpus[idx + t]) contexts.append(cs) return np.array(contexts), np.array(target) class SimpleCBoW: def __init__(self, vocab_size, hidden_size): V, H = vocab_size, hidden_size W_in = 0.01 * np.random.randn(V, H).astype('f') W_out = 0.01 * np.random.randn(H, V).astype('f') self.layers = [ MatMul(W_in), MatMul(W_out) ] self.loss_layer = SoftmaxCrossEntropy() self.params, self.grads = [], [] for layer in self.layers: self.params += layer.params self.grads += layer.grads self.word_vecs = W_in def forward(self, contexts, target): h0 = self.layers[0].forward(contexts[:, 0]) h1 = self.layers[0].forward(contexts[:, 1]) h = (h0 + h1) * 0.5 score = self.layers[1].forward(h) loss = self.loss_layer.forward(score, target) return loss def backward(self, dout=1): ds = self.loss_layer.backward() da = self.layers[1].backward(ds) da *= 0.5 self.layers[0].backward(da) return None window_size = 1 hidden_size = 5 batch_size = 3 max_epoch = 1000 text = 'You say goodbye and I say hello.' corpus, word_to_id, id_to_word = preprocess(text) vocab_size = len(word_to_id) contexts, target = create_contexts_target(corpus, window_size) target = convert_one_hot(target, vocab_size) contexts = convert_one_hot(contexts, vocab_size) model = SimpleCBoW(vocab_size, hidden_size) optimizer = Adam() params = {'W_in': model.params[0], 'W_out': model.params[1]} grads = {'W_in': model.grads[0], 'W_out': model.grads[1]} losses = [] for epoch in range(max_epoch): idx = np.random.choice(len(contexts), batch_size) contexts_batch = contexts[idx] target_batch = target[idx] loss = model.forward(contexts_batch, target_batch) losses.append(loss) model.backward() optimizer.update(params, grads) if epoch % 100 == 0: print(f"Epoch {epoch}, Loss: {loss}") plt.plot(range(max_epoch), losses) plt.xlabel('Iterations') plt.ylabel('Loss') plt.title('NeuralNetBased SimpleCBoW learning') plt.show()
Epoch 0, Loss: 1.9462978261694328
Epoch 100, Loss: 1.9321428971895667
Epoch 200, Loss: 1.7710999737188786
Epoch 300, Loss: 1.6278913730802829
Epoch 400, Loss: 1.450536580343979
Epoch 500, Loss: 1.0928482919036278
Epoch 600, Loss: 1.2757025312900494
Epoch 700, Loss: 1.3082466101926877
Epoch 800, Loss: 0.8394400488379065
Epoch 900, Loss: 0.7968389886601764
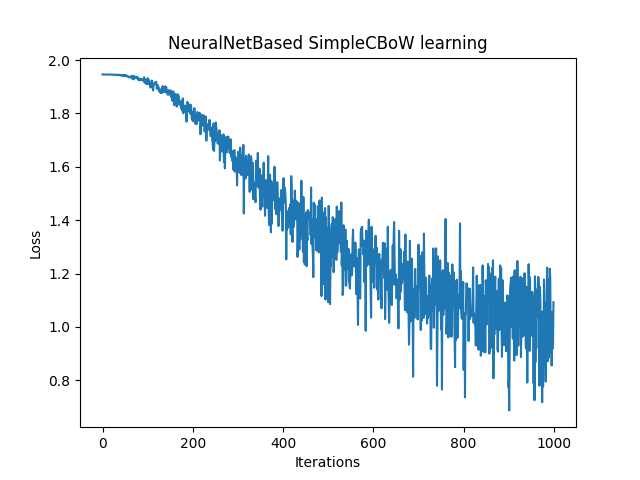
■おわりに
今回はCBoWを用いたニューラルネットワークを実装し、簡単なコーパスを学習させてみました。見慣れた損失とイタレーションのグラフからモデルが学習できている様子が確認できます。
■参考文献
- Andreas C. Muller, Sarah Guido. Pythonではじめる機械学習. 中田 秀基 訳. オライリー・ジャパン. 2017. 392p.
- 斎藤 康毅. ゼロから作るDeep Learning Pythonで学ぶディープラーニングの理論と実装. オライリー・ジャパン. 2016. 320p.
- 斎藤 康毅. ゼロから作るDeep Learning② 自然言語処理編. オライリー・ジャパン. 2018. 432p.
- ChatGPT. 4o mini. OpenAI. 2024. https://chatgpt.com/
- API Reference. scikit-learn.org. https://scikit-learn.org/stable/api/index.html
- PyTorch documentation. pytorch.org. https://pytorch.org/docs/stable/index.html
- Keiron O’Shea, Ryan Nash. An Introduction to Convolutional Neural Networks. https://ar5iv.labs.arxiv.org/html/1511.08458
- API Reference. scipy.org. 2024. https://docs.scipy.org/doc/scipy/reference/index.html